Unstructured data is a critical enterprise cybersecurity issue today. And the volume of this data is soaring. But it can be managed fairly easily, as long as organizations think through their data objectives—especially in terms of compliance. That’s one of many conclusions from the experts who participated in our recent webinar Governing Unstructured Data: Microsoft-Enabled Data Classification and Protection.
As Marvin Tansley, Edgile Information Practice Partner explained, the people, process and technology issues across an enterprise can be effectively resolved by breaking down the data—and the company’s various needs for that data—into categories and then assigning appropriate risk levels for all of that data so the business needs are understood.
We typically start the process by breaking the data challenges into a series of steps:
- Engage all business groups to identify data repositories and define existing data classifications.
- Define data and labeling models, risk classifications, and remediation plans.
- Scan all data for each business group and report the risk exposure dealing with file inventory and risk classifications.
- Review, prioritize and execute plans for remediations per risk classification.
The goal of this process is to keep data classification simple and expand tasks based only on potential risk impact.
The most effective data governance programs do not set out to address all risks in all systems. It’s more effective to prioritize the most likely places for risk to reside as well as the sensitive data with the highest potential impact. A few routine tactics Edgile finds helpful include:
- Use existing metadata scans.
- Filter for regulatory data domains.
- Interview users to better understand people, process, technologies and business purposes.
- Identify gaps in processes and technologies.
In the webinar, Raul Andaverde, Edgile Director, detailed how Edgile partner Microsoft facilitates these processes in a sophisticated and automated fashion. “What you’ll find is that once you have implemented these processes, you’ll have created what Microsoft calls sensitive information types,” noted Anderverde. “These information types coupled with automated algorithms help you detect the sensitive data within these documents. Data remediation and detection method refinements then must become an ongoing effort. Not only are you creating a data inventory but doing refinements ensures that your data discovery contains true positives while bringing down false positives.”
An example that shows the importance of false positive reduction is flagging Social Security numbers—a pretty common ask.
“There are times when organizations find or store data using different methods, and either the system will not pick up the Social Security number format or it starts to look at other documents that may contain similar patterns and you just get a bunch of false positives,” stated Andaverde. “You start figuring out that because this organization stores this data in this way, you need to refine your detection method.”
To hear more about false positives and many other issues involving the proper handling of unstructured data, please watch the webinar.
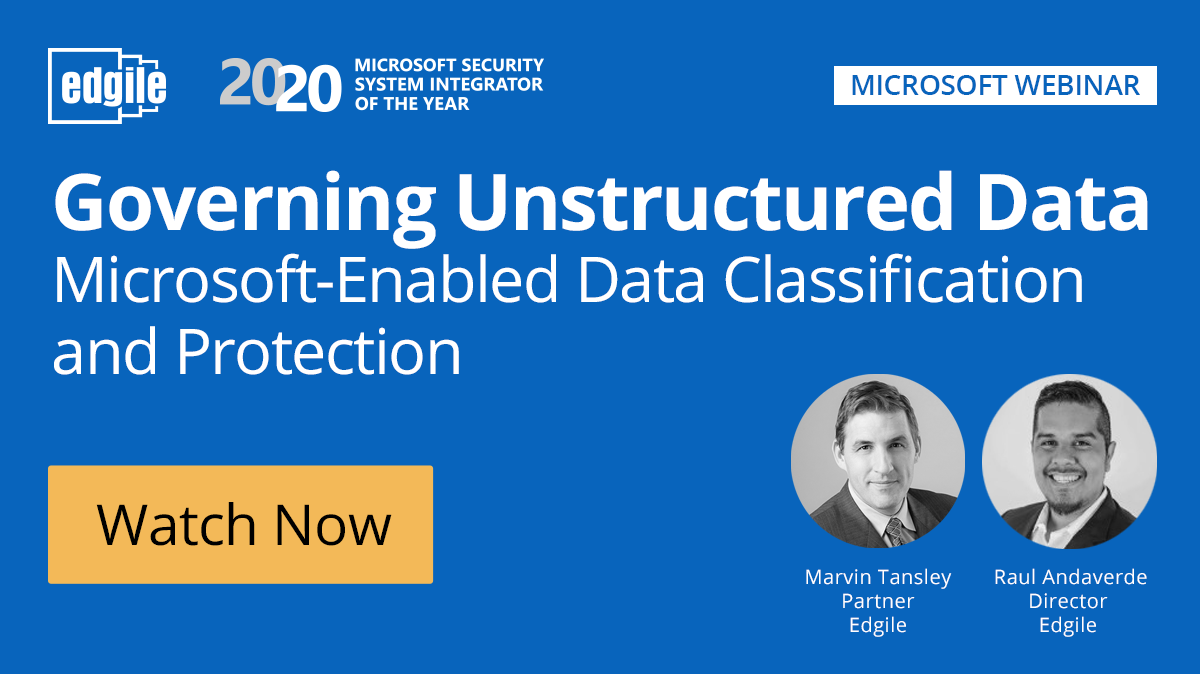